Ventilators, COVID, and Secondary Infection?
What's going on with the recent ventilator story, and did a majority of COVID patients actually die from secondary infection?
For a tl;dr on this study- the results don’t appear to actually suggest increased mortality in those who experience secondary bacterial infections via intubation (VAP), in contrast to remarks made by many that this study actually notes that. There’s an issue in assessing and interpreting information, and it’s quite apparent this failure has happened with respect to this study. Furthermore, the remarks with respect to the lack of cytokine storms is rather controversial, but keep in mind that cytokine storm refers to a specific pathology and can’t be used as loosely as it has in common usage. In short, cytokine storm is not the same as elevated cytokine levels.
Several people have begun to circulate a recent article on ventilators and outcomes in COVID patients.1
A few that I have seen recently include Brian Mowrey’s recent post, as well as Pete Lincoln’s.
Since the beginning of the pandemic there have been several remarks that ventilators may have made outcomes worse for COVID patients and may have contributed in some manner to the death rate.
As the pandemic marched onward, several critics have argued that some of these deaths may have been a result of secondary bacterial infection. This hypothesis has gained some traction, and a while back I proposed a hypothetical model of secondary bacterial infection via gut dysbiosis:
In hindsight I would argue that the model has serious issues- several of the articles included make use of the phrase “cytokine storm”, and I’ll provide an explanation as to why this phrase was used improperly in my review. It also didn’t look closely into the issue of mechanical ventilation and its role in secondary bacterial infection.
Overall, such a study has led to mainstream outlets to cover this story, but doing so in a rather disingenuous way.
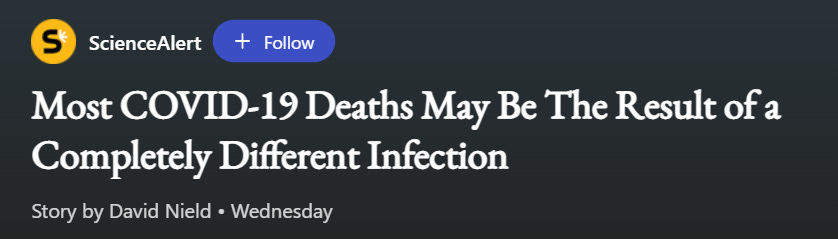
A new analysis suggests that a high percentage of people who required help from a ventilator due to a COVID-19 infection also developed secondary bacterial pneumonia. This pneumonia was responsible for a higher mortality rate than the COVID-19 infection.
So while COVID-19 may have put these patients in the hospital, it was actually an infection brought on by the use of a mechanical ventilator that was more likely to be the cause of death when this infection didn't respond to treatment.
Northwestern University, which many of the authors of the paper are affiliated with, also released an article with respect to this study:
Secondary bacterial infection of the lung (pneumonia) was extremely common in patients with COVID-19, affecting almost half the patients who required support from mechanical ventilation. By applying machine learning to medical record data, scientists at Northwestern University Feinberg School of Medicine have found that secondary bacterial pneumonia that does not resolve was a key driver of death in patients with COVID-19, results published in the Journal of Clinical Investigation.
Bacterial infections may even exceed death rates from the viral infection itself, according to the findings. The scientists also found evidence that COVID-19 does not cause a “cytokine storm,” so often believed to cause death.
By all accounts, the above titles and excerpts act as a bit of a mischaracterization of this study, as both cases above allude to a from perspective when it comes to these mortalities. That is, there’s an assumption here that many of these patients died from the secondary bacterial infection rather than with these infections acting as a contributing factor.
Nonetheless, many people have weighed in on this topic, with the main point being an argument that secondary infection was a big driver of deaths while also discussing the findings on cytokine storms not being apparent in these intubated patients.
These will be discussed below with some additional context provided.
Defining a few terms
There’s a lot going on with this study, and I won’t argue that I have figured out everything that it has displayed. Because of how confusing the information is it’s important to define a few terms that appear throughout the paper. Note that this section is for reference and can be skipped over if needed.
SCRIPT
The results of this study come from an endeavor titled Successful Clinical Response in Pneumonia Therapy, or SCRIPT.
The intent of this study was to examine approaches in dealing with various forms of pneumonia, including those derived from an infection, ones acquired through hospitalization (hospital-acquired pneumonia, or HAP), or ones acquired through intubation (ventilator-associated pneumonia, or VAP):
The goal of this study is to iteratively identify, validate and refine biomarkers to predict clinical failure, and identify novel targets for therapy, beyond traditional antibiotics, for patients with severe pneumonia requiring intubation, including severe community-acquired pneumonia (CAP), hospital-acquired pneumonia, and ventilator-associated pneumonia (HAP/VAP). We hypothesize that factors within the alveolar microenvironment in many patients with pneumonia prevent the active process of pneumonia resolution.
In order to carry out their study, samples of the lungs were carried out via bronchoalveolar lavage (BAL), in which a saline fluid is dispensed into a region of the lung and quickly taken back up. The sample is then analyzed for various determinants such as microbes or other clinical features:
To address this challenge, we have assembled a talented group of investigators in the Successful Clinical Response In Pneumonia Therapy (SCRIPT) Systems Biology Center. We will leverage our routine clinical practice of safe alveolar sampling in mechanically ventilated patients with pneumonia with repeated non-bronchoscopic bronchoalveolar lavage (NBBAL) sampling over the course of pneumonia. From this fluid, we will combine flow cytometry with multi-omic technologies (cell population-specific transcriptomics, epigenomics, shotgun microbiome sequencing, and pathogen-specific sequencing). Our systems scientists will integrate these genomic data with robust clinical -omics with the goal of identifying biomarkers that can be prospectively tested in patients and causally evaluated in humanized mouse models.
The above excerpt notes that NBBAL, a less invasive method of lung sampling, would be carried out rather than the typical method of BAL. However, either approaches may appear in the article (in general, it appears BAL is mentioned, although this may reference either techniques).
Gao, et al. notes that BAL samples were taken periodically, although the exact number doesn’t appear to be described with only scant details provided in the study relating to supposed cases of pneumonia:
ICU physicians at NMH routinely obtain bronchoscopic or non-bronchoscopic BAL samples from mechanically ventilated patients whenever pneumonia is suspected (47). In SCRIPT, patients were screened for enrollment when the clinical team decided to perform the first BAL procedure. For all BAL samples, NMH clinical laboratories perform quantitative bacterial culture and antimicrobial susceptibility testing.
The language here is ambiguous, and will be a point of contention later on.
The only outline of an example of BAL sample collection is noted in the following timeline of one patient, with lines noting BAL sample collection:
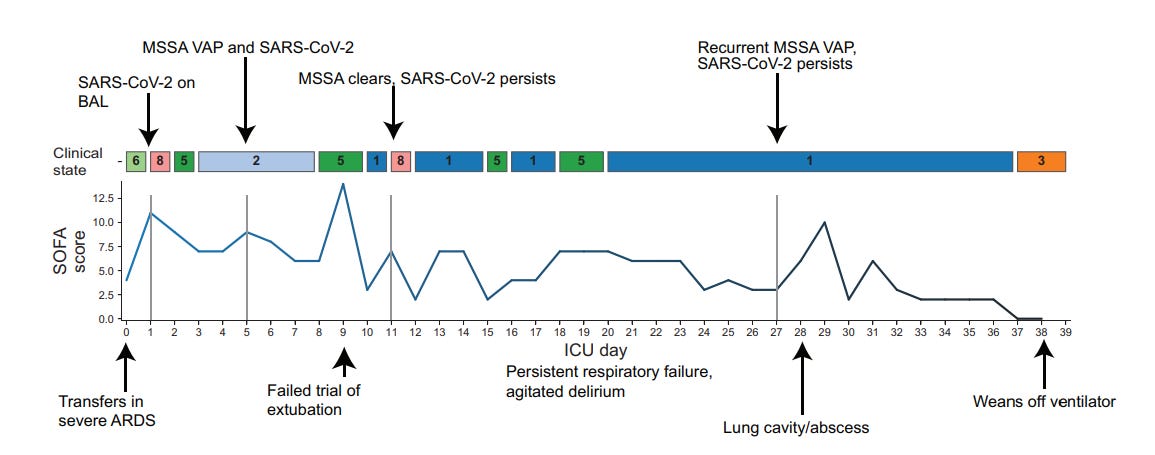
Ventilator-associated pneumonia (VAP)
VAP is a unique form of pneumonia, generally characterized by pneumonia that occurs after 48 hours of being placed onto a ventilator. The cause of VAP is generally attributed to a bacterial infection, mostly relating to S. aureus.
A review article from Kohbodi, et al.2 notes the following:
Ventilator-associated pneumonia is typically bacterial and from a single organism. However polymicrobial infections are increasing. In a large retrospective review done in the ICU settings of three hospitals, the microbiology was the same across adult and pediatric hospitals. The most common organisms were Staphylococcus aureus (28.4 %), Pseudomonas aeruginosa (25.2 %), and other gram negatives (26.6%). [4][5]
Artificial airways become colonized with pathogenic bacteria soon after intubation or tracheostomy, and the major pathogens include both gram-positive and gram-negative bacteria: S. aureus (including MRSA), P. aeruginosa, and Klebsiella and Enterobacter species. Patients in a NICU have a risk of Enterococcus species and group B Streptococcus as well. Other pathogens include the Streptococcus, Enterobacteriaceae, and Acinetobacter species. Anaerobic bacteria are an uncommon cause of Ventilator-associated pneumonia but can play a role in polymicrobial infections, particularly when pneumonia is due to aspiration. Nosocomial viruses and fungi are rare causes of pneumonia in immunocompetent hosts.
In addition, early pneumonia (less than 4 days after admission to the hospital) is most likely from antibiotic-sensitive community-acquired organisms, and late pneumonia (more than 4 days) is more likely due to antibiotic-resistant organisms. However, this distinction is not helpful in children who are frequently admitted to the hospital.
As noted above, ventilation may allow bacteria from other regions of the body including the throat, skin, or even from the hospital to make their way into the upper respiratory tract and cause a secondary infection.
It’s important to remember that everyone within the Gao, et al. study had a case of pneumonia prior to VAP (aside from some who were found to not have pneumonia, and were later turned into controls).
CarpeDiem AI Software
In order to assess their data and check for correlations the SCRIPT researchers used an AI called CarpeDiem to categorize patients based on their information:
To disentangle the effect of VAP on outcomes over the course of the ICU stay, we developed a machine learning approach, CarpeDiem, which clustered individual patient-days in the ICU using clinical parameters extracted from the electronic health record (EHR). Because key clinical data fed the CarpeDiem algorithm, these clusters represented clinical states that were differentially associated with hospital mortality. The CarpeDiem framework allowed us to examine transitions between clinical states associated with favorable (lower mortality) or unfavorable (higher mortality) outcomes.
Keep in mind that the use of AI is heavily reliant on the data you provide it. It’s an example of the results you get out being entirely dependent upon the data you put in. In that regard, the correlations here should be looked at with some skepticism as any exclusion/inclusion of data would inherently bias the results.
Because of this issue, not much will be reviewed with respect to CarpeDiem, as the use of AI comes with the consequence of creating a fishing expedition (i.e. reading into the data and inferring too much with respect to any correlation).
As an example, CarpeDiem is noted to provide a model based on the following factors (emphasis mine):
For all 12,495 ICU patient-days in the cohort, we extracted clinical data from the EHR describing 44 key clinical parameters, including flags for organ failures requiring mechanical support (e.g., mechanical ventilation, renal replacement therapy, and ECMO), continuously recorded clinical parameters (e.g., vital signs and doses of norepinephrine), and commonly measured laboratory values (Supplemental Figure 3A). Variables used to calculate the SOFA score are a subset of these parameters. Importantly, patient-intrinsic variables (e.g., demographics, BMI, tracheostomy, and diagnosis), biochemical and microbiological analyses of BAL fluid studies, and adjudication of VAP episodes were not included in the model.
This is further elaborated on within the Discussion section when describing limitations in this study:
Fourth, CarpeDiem uses a limited number of parameters to define clinical states, potentially neglecting important determinants of outcome and information that might be found in missing data (e.g., reduced monitoring and ordering of laboratory tests as patients improve or move toward comfort-focused care). Similarly, intermittently measured biomarkers associated with outcomes, for example those used by Calfee et al. to define hyper- and hypo-inflammatory states in the ICU, are incompletely represented in CarpeDiem (46)
Because these key factors have been excluded from the analysis there’s going to be several flaws in interpreting the correlative power of some of CarpeDiem's results.
Clinical States
Throughout the study several references are made to “clinical states”. Clinical states aren’t a standard term found in the literature. Rather, clinical states are arbitrary categorizations constructed by CarpeDiem in order to organize patient data in a meaningful way. There are 14 clinical states in total constructed by CarpeDiem, and the criteria for each clinical state is rather different. The clinical states are numbered based upon mortality rate, with 1 having the fewest mortalities and 14 having the highest.
However, what’s important to remember is that each clinical state doesn’t inherently progress into the next one. For instance, it’s not uncommon to see that patients will jump from a clinical state of, say, 7 to 12 (I chose these at random so not something that may be seen in the data). Again, each clinical state is recognized with a different criteria, with a few relating to hypoxemia (low oxygen in the blood) or severely reduced respiratory function.
An example is clinical state 12, which is defined in the study as follows:
For example, clinical state 12 represents patient-days with very severe respiratory failure (mostly days spent receiving ECMO support), moderately high levels of sedation, an intermediate level of shock without substantial renal failure, and with relatively stable ventilator settings.
The other clinical states don’t appear to be described, and information on these clinical states require reaching out to the authors for additional information, so as of now the categorization for the other clinical states are generally unknown.
Also, the study makes reference to transitions, which are when one person transitions from one clinical state to another.
Overall, just remember to not get bogged down and read too deeply into the organization of clinical states when looking at figures such as the one below:
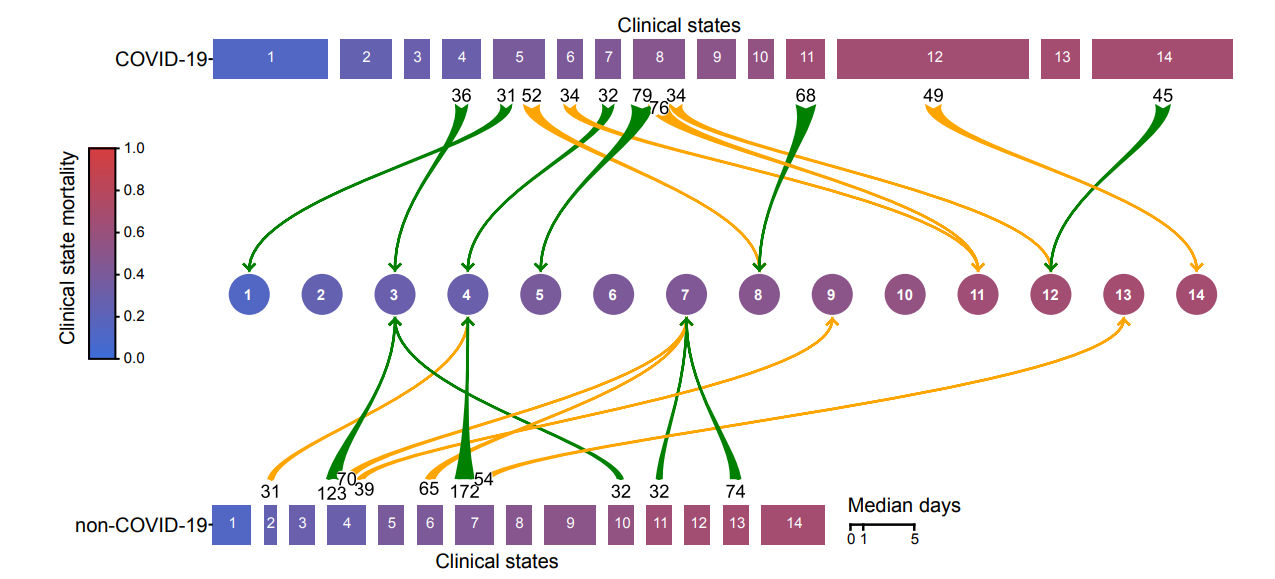
In regards to Secondary Infection and Cytokine Storm
With respect to this study we’ll try to tackle the two topics above. Many people have already discussed these topics such as Mowrey And Lincoln cited above, but I’ll further elaborate on this finding.
To help provide a summary of the findings the researchers provided this graphical abstract:
Overall, I’ll argue that the study has serious issues in outlining their data. There’s a ton missing here, including which bacteria were cultured from BAL samples. The timing of BAL collection is up in the air, and the study also doesn’t make it clear early on how many patients actually experienced an episode of VAP, whether in the COVID group or the other groups (you have to dig into the actual body to find a reference to VAP episodes).
The lack of organization makes the study rather difficult to read, and I won’t say that I have it figured out yet. This is just a forewarning for those who would try to take a gander for themselves.
Secondary Bacterial Infection (VAP) did not appear to contribute to a large portion of deaths, contrary to what has been reported.
This study looked at retrospective data from over 585 patients starting from 2018 and up to 2022.
Out of the included patients 190 had a suspected case of SARS-COV2 pneumonia, with the overall breakdown described below:
Of 601 patients enrolled in SCRIPT between June 2018 and March 2022, 585 had an adjudicated pneumonia category and clinical endpoints at the time of analysis (Figure 1): 190 had COVID-19, 50 had pneumonia secondary to other respiratory viruses, 252 had other pneumonia (bacterial), and 93 were initially suspected of having pneumonia yet were subsequently adjudicated as having respiratory failure unrelated to pneumonia (non-pneumonia controls).
Based on prior evidence of secondary bacterial infection, as suggested by post-hoc assessment of the 1918 Spanish Flu outbreak which noted a possible high degree of bacterial infection, and paired with suspected cases of secondary infection based on autopsy reports, the authors wanted to investigate the extent to which intubation of SARS-COV2 patients may have led to secondary bacterial infections (VAP), and whether this contributed to outcomes in some way, such as with respect to mortality.
Note that all of the participants in this study had BAL samples collected at the time of admission into the study, and were also intubated.
Now, when looking at VAP episodes there was a discrete difference between COVID patients and non-COVID patients, in that around 57.4% (~109 patients) of COVID patients had at least one episode of VAP:
Overall, 35.5% of patients in the cohort developed at least one episode of VAP during their ICU stay (25.0% among patients without COVID-19 compared to 57.4% among patients with COVID-19, p < 0.001) (Figure 7A). 8.7% of patients in the cohort experienced more than one episode of VAP (3.5% among patients without COVID-19 compared to 19.5% among patients with COVID-19, p < 0.001) (Figure 7B). Mortality in patients with VAP has been reported to increase substantially with each ensuing episode, approaching 100% in patients with three or more episodes (36).
Be careful with respect to the last sentence. Although mortality was near 100% in those with additional VAP episodes, the overall number of those experiencing additional VAP episodes was much lower relative to the other groups (noted in Figure 7C below):
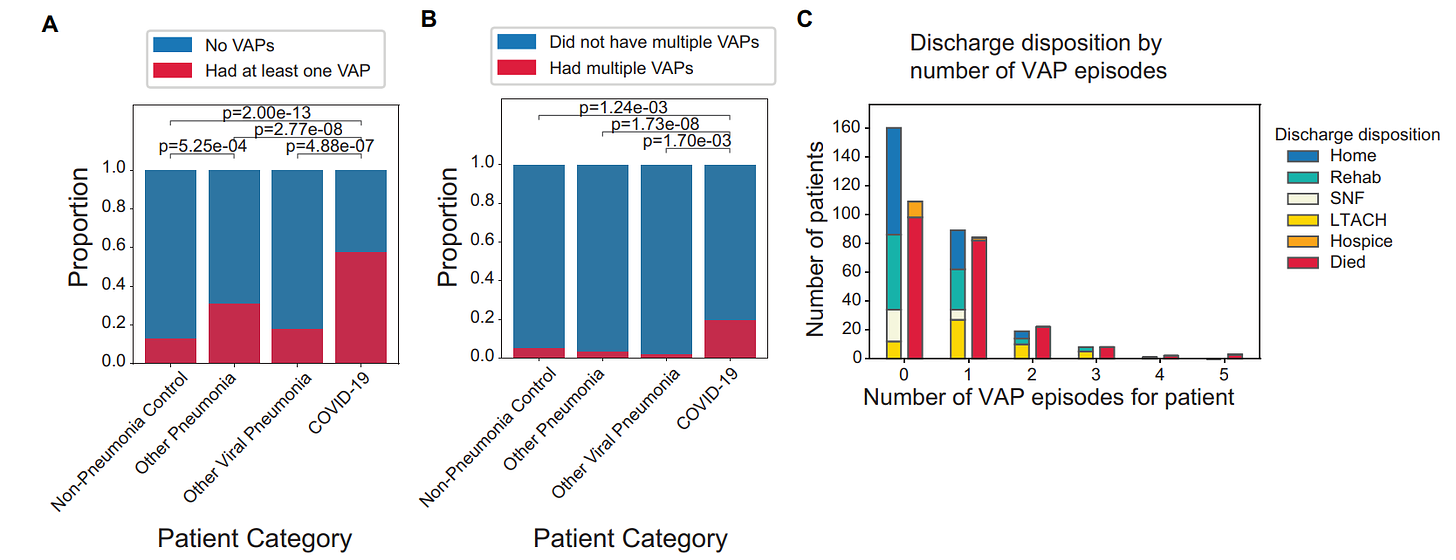
What’s interesting is that mortality across all 4 groups appear rather similar:
So here COVID patients experienced greater episodes of VAP while having a similar mortality rate as the other groups.
There are a few reasons for this, as it’s possible that the other groups may have died prior to experiencing a VAP episode and thus may result in the relatively low rates.
With respect to COVID, a clearer assumption may suggest that severe lung tissue damage from SARS-COV2 may greatly increase the risk of VAP, as the lung environment may be far more susceptible to colonization from other bacteria.
This is where the clinical state transitions comes in.
Here, it’s noted that clinical state transitions for COVID patients were relatively lower with respect to the other pneumonia groups. However, COVID patients appear to have shuffled between clinical states with respect to their level of hypoxemia, with clinical states associated with hypoxemic respiratory failure being comprised predominately of COVID patients:
Clusters that were enriched in days from patients with COVID-19 had higher respiratory severity scores (Figure 3, Figure 4, and Figure 5D), illustrating that patients with COVID-19 spent a disproportionate amount of time in clusters characterized by hypoxemic respiratory failure. Time spent in clinical state 12, characterized by severe hypoxemic respiratory failure, accounted for 29.9% of the difference in ICU LOS experienced by patients with COVID-19. Overall, since some clusters were deficient in patients with COVID-19, time spent in the four clinical states that were significantly enriched in patients with COVID-19 accounted for over 100% of the difference in ICU LOS between patients with and without COVID-19.
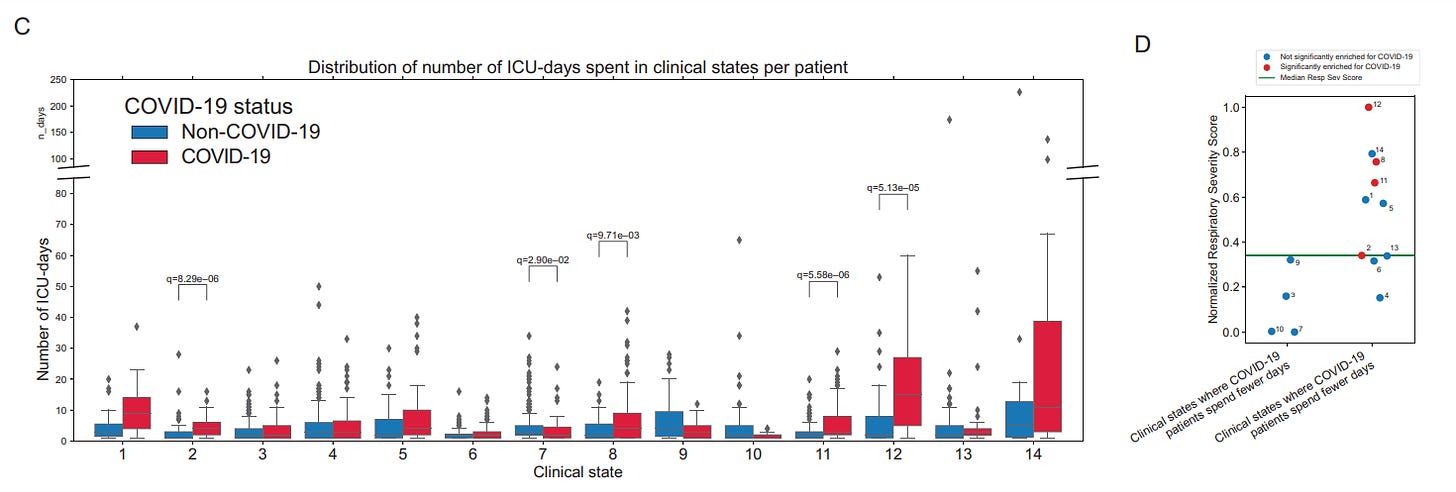
Taken altogether, the evidence actually doesn’t argue what has been remarked on by many people, in that secondary bacterial infections are not leading to a large portion of deaths for COVID patients.
It’s quite clear that many of these individuals are already in various states of severe respiratory distress and failure as noted by the clinical states and relative mortality rates, making these people more at risk of death irrespective of from SARS-COV2 or a bacterial infection.
This is one of the arguments I agree with Brian on, in that the evidence cannot suggest a high degree of death related to secondary infection as people have taken to report. And as Pete mentioned, in those who died from with secondary bacterial infection these infections were unresolved (patients were either in an indeterminate state of VAP, or VAP was not cured).
This is noted in both Figure 8A & B:
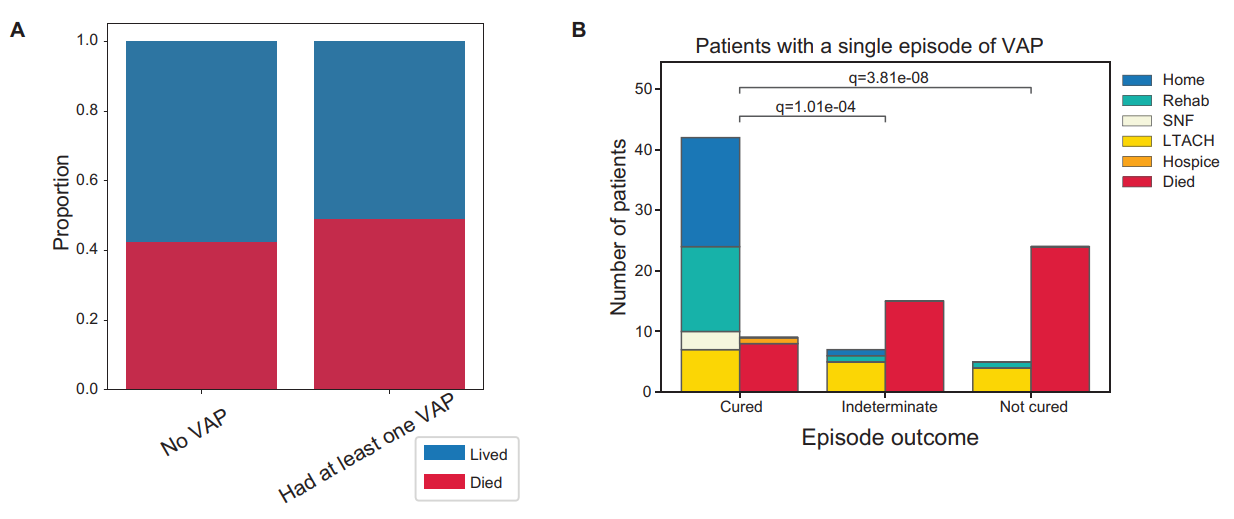
The authors themselves note that this does not appear to be the case, which is rather strange given their remarks about secondary bacterial infections:
Overall, mortality was not significantly different in patients who developed VAP compared to those who did not (Figure 8A).
This is a case of reporting that glosses over (don’t sue me Brian!) some of the nuance, and instead creates a headline not substantiated by the actual evidence.
The fact the researchers themselves have made these remarks in various outlets is rather surprising, and goes against their own findings. It’s not necessarily the secondary infection that is cause for concern, but the fact that many patients require ventilation. Upon ventilation, the secondary concern may be the secondary bacterial infection, although the researchers don’t provide any insights into why some patients were not able to resolve their VAP episode.
This is, again, an issue with the lack of data provided by the researchers themselves.
Cytokine “storm” vs Cytokine elevation
As this article has run on for too long, I will comment on the idea of cytokine storm a bit here.
I have used the term cytokine storm in prior articles, generally as a response to greatly elevated cytokine and inflammatory markers with respect to certain diseases or pathologies.
However, this use of cytokine storm is rather improper, and likely owes to the use of the word “storm” in much the same ways that “sin” has damaged interpretations of Original Antigenic Sin.
Because of this the use of “cytokine storm” has run into issues of inconsistency, with several scientists using the term in different manners.
Fajgenbaum, D. C., & June, C. H. 3 makes the following remark with respect to ambiguous use of cytokine storm:
No single definition of cytokine storm or the cytokine release syndrome is widely accepted, and there is disagreement about how these disorders differ from an appropriate inflammatory response. The National Cancer Institute’s definition, based on the Common Terminology Criteria for Adverse Events (CTCAE), is too broad, since the criteria for an inflammatory syndrome can also apply to other physiological states, and the definition of the American Society for Transplantation and Cellular Therapy is based on criteria that focus too specifically on iatrogenic causes of cytokine storm alone.7 Although cytokine storm is easy to identify in disorders with elevated cytokine levels in the absence of pathogens, the line between a normal and a dysregulated response to a severe infection is blurry, especially considering that certain cytokines may be both helpful in controlling an infection and harmful to the host. The interdependence of these inflammatory mediators further complicates the distinction between a normal and a dysregulated response.
This has led to the misuse of cytokine storm to reference any manner of cytokine elevation as I have mistakenly done in the past, because how exactly would one quantify a “storm”?
Terms in science can be considered a term of art, wherein their use with respect to a certain topic may be specific. This is different than colloquial use of a word, which may infer something different.
In this regard, the use of cytokine storm appears to have differed with respect to the actual use in science.
As Fajgenbaum, D. C., & June, C. H. notes, and has been described in various pieces of literature, cytokine storm is generally associated with multi-organ dysfunction and the possibility of failure:
Cytokine storm is an umbrella term encompassing several disorders of immune dysregulation characterized by constitutional symptoms, systemic inflammation, and multiorgan dysfunction that can lead to multiorgan failure if inadequately treated (Figure 1). The onset and duration of cytokine storm vary, depending on the cause and treatments administered.7 Although the initial drivers may differ, late-stage clinical manifestations of cytokine storm converge and often overlap. Nearly all patients with cytokine storm are febrile, and the fever may be high grade in severe cases.8 In addition, patients may have fatigue, anorexia, headache, rash, diarrhea, arthralgia, myalgia, and neuropsychiatric findings. These symptoms may be due directly to cytokine-induced tissue damage or acute-phase physiological changes or may result from immune-cell–mediated responses. Cases can progress rapidly to disseminated intravascular coagulation with either vascular occlusion or catastrophic hemorrhages, dyspnea, hypoxemia, hypotension, hemostatic imbalance, vasodilatory shock, and death. Many patients have respiratory symptoms, including cough and tachypnea, that can progress to acute respiratory distress syndrome (ARDS), with hypoxemia that may require mechanical ventilation. The combination of hyperinflammation, coagulopathy, and low platelet counts places patients with cytokine storm at high risk for spontaneous hemorrhage.
There’s a lot going on here, but there’s an apparent assumption that cytokine storms are related to distress of various organ systems.
It’s this definition that Gao, et al. uses in their study, and described by co-author Benjamin Singer in the Northwestern article:
“The term ‘cytokine storm’ means an overwhelming inflammation that drives organ failure in your lungs, your kidneys, your brain and other organs,” Singer said. “If that were true, if cytokine storm were underlying the long length of stay we see in patients with COVID-19, we would expect to see frequent transitions to states that are characterized by multi-organ failure. That’s not what we saw.”
This is inferred based on the clinical states outlined above, in which many COVID patients resided in clinical states associated with hypoxemic respiratory failure rather than multi-organ dysfunction/failure.
Of course, one caveat to this assumptions is figuring out how each clinical state is categorized, and whether there is overlap or exclusion of factors such as respiratory failure within multi-organ dysfunction categorization.
The researchers did not take any measures of inflammatory biomarkers, so not much can be inferred with respect to quantitative data.
However, it’s also true that the researchers are following a proximal feature of cytokine storm, in that the lack of multi-organ dysfunction clinical state categorization may suggest a lack of cytokine storm occurring with these patients.
This is where the discrepancy between how cytokine storms are used and defined, as in Gao, et al. relative to how people infer cytokine storms.
This is why it’s important that researchers define terms, and use terms in a manner that is appropriate or common within the literature. There’s a clear distinction in the discussing inflammation and cytokine release vs an actual “cytokine storm”, which carries a certain course of events and pathology.
Tl;dr…sort of…
Overall, I find that this study is leaving me wanting of more data. The study appears to rely far too much on CarpeDiem and in that case the use of this AI may lead to inferences that may not actually be true.
Keep in mind that CarpeDiem assesses the data it’s provided, and if data is missing then it may produce correlative red herrings. When comparing a different dataset (Mimic-IV), the researchers noted that the clinical states CarpeDiem constructed were actually different, and hence again emphasizes that the categorization and assessment of CarpeDiem is fully reliant on the data it is provided:
While these results support the generalizability of the CarpeDiem approach, the clinical states observed in the MIMIC-IV cohort were not identical to those in the SCRIPT cohort. This observation might be expected as, for example, MIMIC-IV had very few patients receiving ECMO, underscoring the concept that clinical states cannot be assigned a priori in a given cohort.
When it comes to the notion that many people died from a secondary bacterial infection, the results here aren’t exactly substantiated by the data. Rather, the outcomes seem comparable between those who had a VAP episode and those who didn’t. Since no background non-intubated patient data was provided, there’s no way to assess the discrepancy between intubation vs non-intubated outcomes, again adding to the notion that such conclusions cannot be drawn given the data provided.
The arguments over cytokine storm can be considered rather controversial, although one can argue that the inferences made by Gao, et al. follow along with typical descriptions of cytokine storm, although additional data would provide better assumptions.
At the end of the day, I don’t think this study offers as much as I assumed when entering. The fact that many people have reported conclusions not substantiated by the study is concerning- it suggest an issue of self-reference on both Substack in news outlets, as well as possible lack of reading of the studies.
Substack is my main source of income and all support helps to support me in my daily life. If you enjoyed this post and other works please consider supporting me through a paid Substack subscription or through my Ko-fi. Any bit helps, and it encourages independent creators and journalists such as myself to provide work outside of the mainstream narrative.

Gao, C. A., Markov, N. S., Stoeger, T., Pawlowski, A. E., Kang, M., Nannapaneni, P., Grant, R. A., Pickens, C., Walter, J. M., Kruser, J. M., Rasmussen, L. V., Schneider, D., Starren, J., Donnelly, H. K., Donayre, A., Luo, Y., Budinger, G. R. S., Wunderink, R. G., Misharin, A. V., & Singer, B. D. (2023). Machine learning links unresolving secondary pneumonia to mortality in patients with severe pneumonia, including COVID-19. The Journal of clinical investigation, e170682. Advance online publication. https://doi.org/10.1172/JCI170682
Kohbodi GNA, Rajasurya V, Noor A. Ventilator-associated Pneumonia. [Updated 2022 Sep 10]. In: StatPearls [Internet]. Treasure Island (FL): StatPearls Publishing; 2023 Jan-. Available from: https://www.ncbi.nlm.nih.gov/books/NBK507711/
Fajgenbaum, D. C., & June, C. H. (2020). Cytokine Storm. The New England journal of medicine, 383(23), 2255–2273. https://doi.org/10.1056/NEJMra2026131
If million of people die a small percentage may still be “many”...
...that is my concern the attempt to suggest some deaths due to secondary bacterial infection didn’t amount to the vague term “many”
It definitely wouldn't surprise me but as you know, they can NEVER ADMIT THEY WERE WRONG. Lets sing along to the new covid anthem: https://youtu.be/C4WN30VKgOk