Infection Insights from a COVID Longitudinal Surveillance Study
Evidence of the viral dynamics at the early stages of infection, as well as longevity of antibodies and T-Cell responses.
Edit: The link for one of the sections linked to this article and not the proper section (Humoral Immunity Kinetics section). The proper link was added.
Edit 10/20/2022: I added some additional clarification to the asymptomatic case as the researchers comment that the asymptomatic infection was likely caused by two family members with mild infection. The initial comments may have been interpreted to suggest that the two family members may have been the reason for the prior immunity. The change can be noted in italics along with a quote from the article.
Most studies on COVID have served as little images or moments in time. They rarely serve to provide a broad timeline of what occurs in the process of getting infected up to when one has long-term, adaptive immunity.
This has left several gaps in knowledge, including understanding which antibodies and cytokines are released, as well to what extent one becomes infected with COVID after the point of infection.
However, an article published in Nature1 may help to fill in some of these gaps.
This is a much-needed study, and although there are quite a few limitations it’s nice to have this sort of evidence.
Note: I will try to include Sections to these longer posts so that it’s easier for people to pick up where they left off. Now that Substack allows us to link to Headers it makes it easier to direct people to different sections.
Table of Contents
Study Overview
We’ll start with the “Plain Language Summary” to wet everyone’s noodle:
Managing the response to the COVID-19 pandemic requires information about how quickly the virus reproduces and the effect on the immune system of the person who is infected. We measured the speed at which SARS-CoV-2 reproduces in unvaccinated individuals at various timepoints between when they first became infected, and there was no longer any detectable virus present in their bodies. We also measured changes in their immune response. Our findings can be used to develop guidelines for the clinical management of COVID-19 patients and optimize testing procedures to determine whether people are infected with SARS-CoV-2.
This study recruited participants from the Oak Crest Institute of Science, a non-profit located in California. This provided a information on workplace spread of COVID while also allowing the researchers to collect samples, including routine nasal swabs and blood samples.
The recruitment timeframe started on March 23, 2020— around the start of the pandemic, and continued into 2022 providing a nearly 2 year-long study.
Although the researchers recruited up to 152 participants only 9 participants were included in this study.
Unfortunately, this is one of the biggest limitations of this study by providing such a small sample size. The ethnicity data also shows that most of the available data are from predominately female and Hispanic employees.
We’ll make do with what we can get. It’s important to note that almost all participants aside from one was unvaccinated during the time of this study. Participants were also infected at different timepoints as well, meaning that variant will be something to consider in this study as well.
Employees who tested positive during the course of the study were immediately isolated. In this case, 4 of the 9 positive participants were able to be screened routinely and provided information about the early stages of the infection:
Four individuals (subjects 2, 21, 38, and 48) from this positive cohort of nine individuals (Table 1, subjects 2, 21, 38, 48, 63, 82, 83, 84, and 85) were available for direct observation and frequent sampling during the early phase of infection (i.e., proliferation phase).
The labeling of subjects will get confusing as we get into the figures so I’ll try to clarify them when needed.
In any case, nearly all participants presented with symptoms typical of COVID, but no participants required hospitalization.
Now, we’ll get into some of the data.
Viral Load Across Anatomic Compartments
One brave participant decided to provide samples from different “anatomical compartments”—their words, not mine— for nearly two months. These samples include nasal, oral, saliva, and…fecal. So let’s thank the participant who decided to donate stool samples every day for two months for the purpose of science!
This participant, Subject 21, provided daily samples for up to 54 days. Viral load was estimated by RT-qPCR, so bear in mind that PCR is being used as a proxy for actual viral load in this study.
In the charts below the x-axis shows the number of days while the y-axis shows viral load as determined by RT-qPCR:
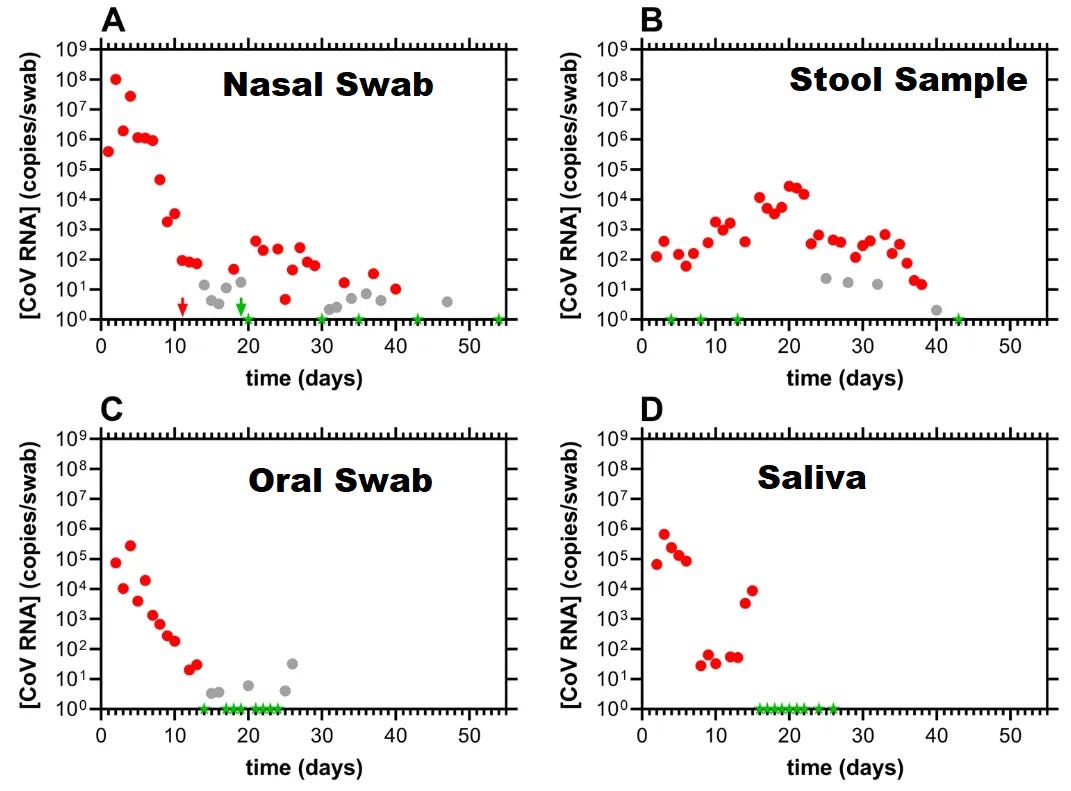
Red dots are for positive results, grey are for inconclusive results (i.e. only amplified for one of the probes but not the other), and green stars are for negative results (i.e. neither probes met the Ct cutoff of 40).
We can see several distinctions in the results:
Nasal and oral samples show immediately high viral loads with a quick decline.
Maximal SARS-COV2 copies (Cmax) for nasal swabs were nearly 370 times higher than oral swabs and saliva, which both had similar Cmax values.
Stool samples show a bell-curve shape where samples showed a slow increase with a Cmax occurring around day 18 before declining, as well as a Cmax value nearly 3800 times lower than nasal swabs.
Remember that these are results in one individual (one brave, brave, individual) and relied on PCR.
However, it does provide some insights into the viral kinetics during the initial stages of the infection, showing viral clearance within the nose and mouth begins days into the initial infection while stool samples showed a wide bell-curve shape suggesting delayed appearance in the intestines. It’s also interesting how long viral clearance (again, using RT-qPCR as a proxy) took within both the nose and stool with both requiring up to 40 days before returning inconclusive/negative results:
Despite this large difference in Cmax, subject 21 remained positive for SARS-CoV-2 RNA for close to 40 days in nasal and stool samples, while positivity in oral samples only lasted for ca. two weeks.
Since PCR was being used here, this doesn’t equate to an active infection up to 40 days in either the nasal passage or in the intestines, so we can’t quite infer what this exactly means aside from the fact that genetic material exists for quite a while in these spaces.
Longitudinal SARS-COV2 Viral Profiles
In another analysis researchers followed 7 of the 9 participants as soon as they turned positive, providing nasal swab samples over the course of several months.
The participants are labeled with their corresponding viral loads for clarity (Sub # short for subject and designated number):
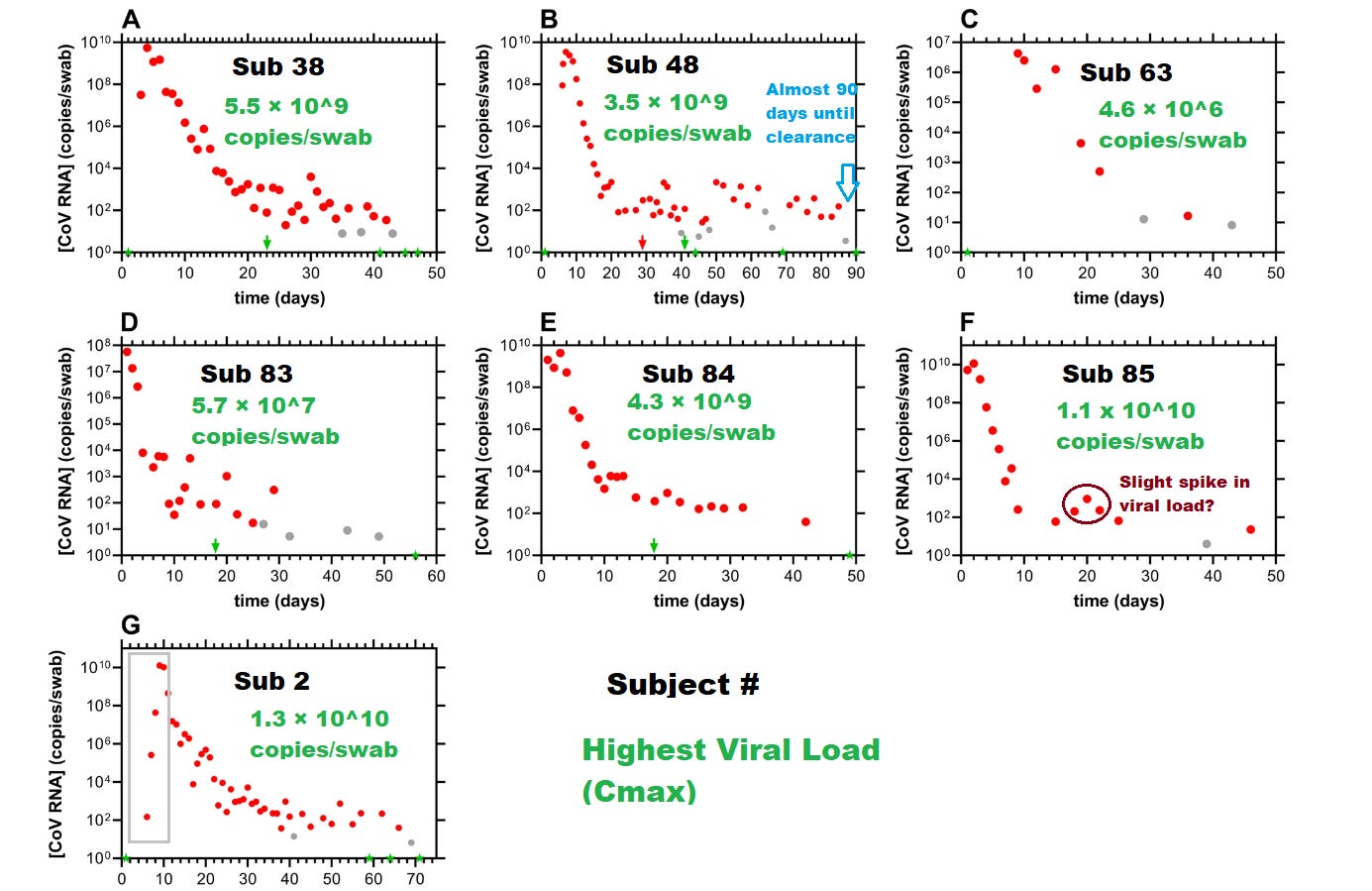
Red, grey, and green data points represent similar results as the ones from the previous “anatomic compartment” section.
Here are a few key findings:
All results, similar to the previous nasal swab result (Subject 21), show a similar trend of rapid decline with a plateau before becoming inconclusive/negative, which we would expect.
Where available, a rapid increase can be seen in viral load after the first positive test (as seen in Subs. 38, 48, and 2), suggesting a rapid proliferation of the virus very early on into the infection.
Time to inconclusive/negative results, an indication by the researchers as the viral clearance phase, varied greatly between participants. For instance, Sub. 83 showed clearance by around Day 30 while Sub. 48 shows positive results almost up to Day 90.
A few participants showed sudden bumps up in viral load, such as Sub. 85 on day 20. The researchers took this to indicate some slight oscillation in viral replication (noted as multimodal decay kinetics), although this may be an artifact from sampling.
Highest viral load varied by several magnitudes across participants (noted in green font in the above figure).
Again, remember that these measured used PCR as a proxy.
There’s also an issue in which data points for many participants are missing, such as Sub. 63 and Sub. 85. The researchers don’t provide any explanation for why there are large gaps in the data.
One possibility could be the time of infection, as PCR testing slowed down between June 9, 2021, and January 3, 2022 to only twice a week. This may indicate fewer data points, but not to the extent that should be seen above.
This should raise a some caution for the extrapolation of some of these participants as the full data isn’t available.
However, given the data from the other participants we can see a clear longitudinal curve in how viral infection of the nasal passage goes, starting with sudden spike in viral load that quickly decays and tapers off before finally being undetectable, generally occurring over the course of weeks/months.
SARS-COV2 Doubling Time
Doubling time, denoted with Td, explains how long it takes for SARS-COV2 viral load to double, providing a hint as to how rapidly the virus may proliferate and quickly it may lead to infection.
Due to nearly daily sampling, researchers were able to collect information for the growth phase of the virus in which viral replication increases, reaches a maximum viral load (Cmax) and then declines, with a clear example being Sub. 2 as indicated with the thin grey box.
Data were available for Subs. 21, 38, 48, and 2, allowing researchers to construct slopes and find individual Td values (using rate equations; refer to Figure 3H shown in the Footnote below for the slope for Sub 22).
Interestingly, 3 of the participants showed similar Td values while one participant (Sub. 48) showed a much higher Td value of nearly 5 hours, suggesting that it took longer for the virus to double in Sub. 48 compared to other participants.
The researchers note that this participant also took the longest to clear the virus, taking up to nearly 90 days:
The Td value for subject 48 was calculated from three timepoints spanning 24 h (Fig. 3B). Subject 48 also took longer to clear the virus than any of the other participants (Fig. 3B).
And can be seen in the figure below showing each participant’s Td value along with a grouped box with a median time around 3.1 hours:
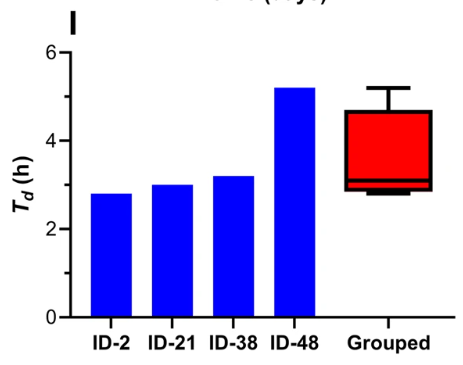
This is one of the first studies to show the doubling of viral load in people.
However, the data here is a bit inconsistent.
Although Sub. 48 had both the highest Td value and the longest clearance time, Sub. 2 appears to have had the second highest viral clearance time yet had the lowest Td value.
This may tell us that there’s no clear correlation between clearance time and viral replication. It’s also important to add that Sub. 48 appears to use 3 timepoints within a 24-hour timeframe, while Sub. 2 use 4 timepoints over the course of 4 days (1 per day).
Usually rate equations are designed to factor in time, but they also would depend on the timing of sampling as closer data points would provide tighter slopes.3 One has to also wonder how many data points were actually used to construct these graphs (did Sub. 38 only use 2 timepoints?).
The consistency between 3 of the sample would at least suggest a doubling time of around 3 hours, however the outlier for Sub. 48, given the timepoint discrepancies, may raise some skepticism.
Either way, the fact that researchers were actually able to collect data during the proliferation phase is commendable, and provides us a window into how quickly SARS-COV2 may replicate in exposed individuals.
Innate Immunity Kinetics
Now we’re getting into some of the really interesting stuff (hopefully the other stuff was interesting!).
Prior studies haven’t shown the full breath of cytokine/chemokine release during the initial stages of a SARS-COV2 infection, as well as the response weeks afterwards.
In short, cytokines and chemokines are signaling molecules responsible for the immunological response during an infection. Their release can lead to recruitment of immune cells, growth and maturation of cells, and provides a broad pro-inflammatory response in times of infection.
Here, researchers collected routine blood samples starting from Day 1 of a positive infection and created individual profiles for 6 of the participants.
Each graph represents a different detectable4 cytokine/chemokine with each participant’s concentration, as noted on the y-axis.
Individual participant’s cytokine/chemokine profile is noted in different colors:
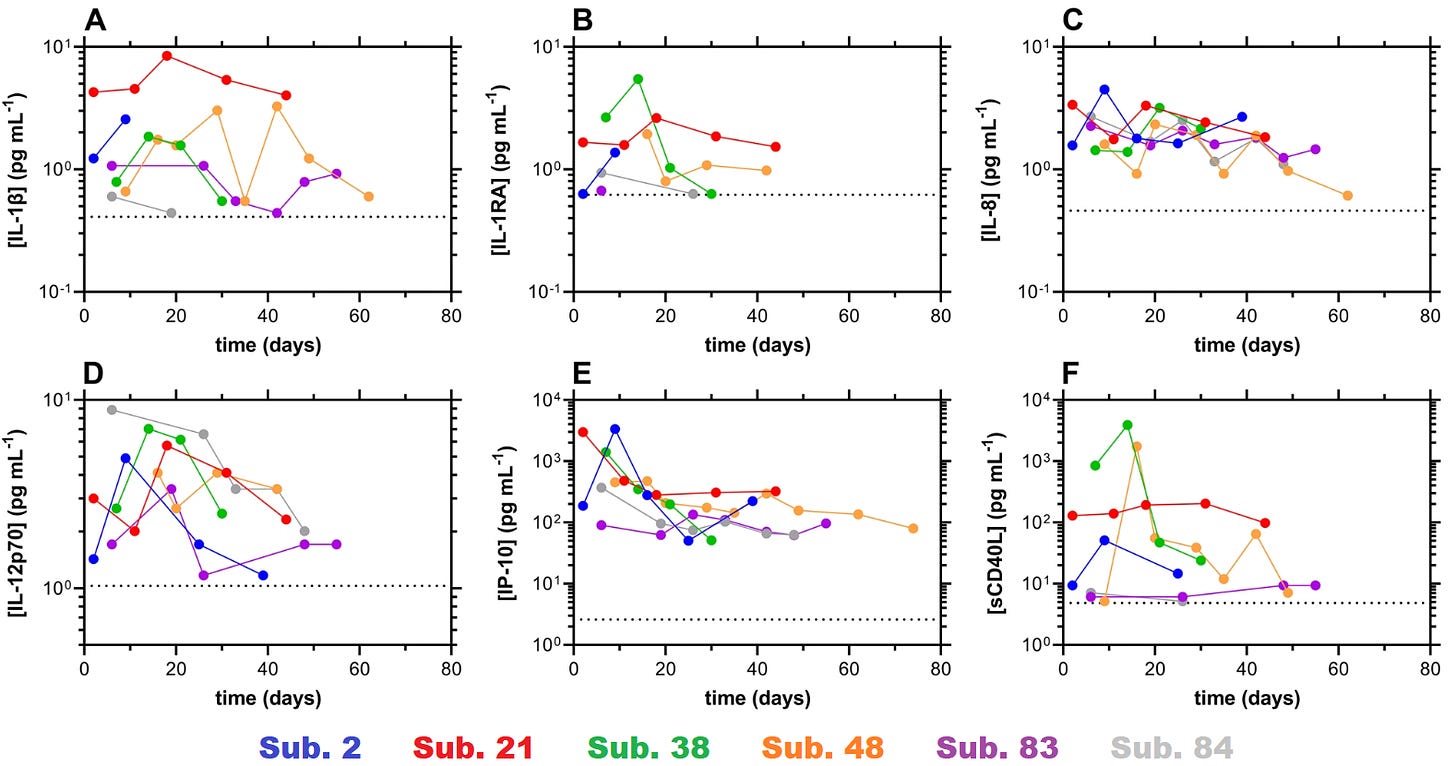
A few noteworthy distinctions can be seen:
Innate immune profiles vary greatly between participants, and can be seen especially with the IL-1β and sCD40L results.
IL-8 response appears to be even across individuals, with a consistent elevated response as compared to other markers measured.
Innate immune marker concentration vary, with IP-10 and sCD40L concentrations appearing the highest among the detectable cytokines/chemokines.
Sub. 21 (in red) shows an elevated profile across all measures, and is especially notable in sCD40L results, which may be suggestive of some type of chronic disease (speculating here).
Doing an assessment for every marker here would be beyond my abilities— more immunologically savvy individuals can pick apart these results more.
However, one interesting result is the soluble form of CD40L (sCD40L), which is released by T lymphocytes and especially platelets during an infection, and has been implicated in thrombosis and other cardiovascular diseases.5
Given that both Sub. 38 and Sub. 48 show a sudden spike in sCD40L, this may be an indication of endothelial dysfunction due to SARS-COV2 infection and may be indicative of possible thrombotic events.
No additional screening or tests were conducted so we’re left in the dark as to how to properly infer these results.
Overall, these results do indicate that people respond to infections differently with cytokine/chemokine release varying widely between participants.
Humoral Immunity Kinetics
Along with the innate immune profile researchers also collected information on antibodies over the course of several months. The antibodies here include IgG, IgA, and IgM. The differences between these immunoglobulins (Ig) can be seen in the Footnote.6
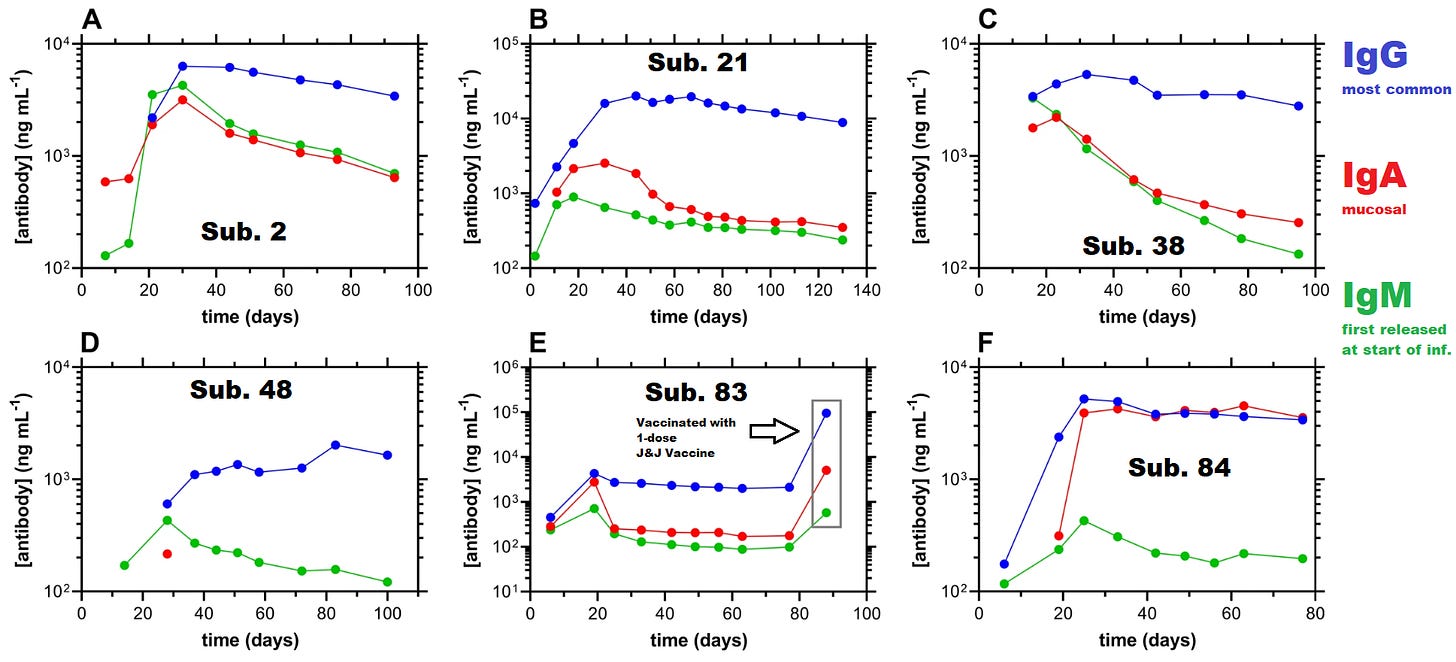
The most important information here is the IgA response, as it’s been argued that the vaccines elicit a predominately IgG-mediated response.
A few noteworthy inferences:
Humoral response varies greatly across all participants.
Sub. 48 showed nearly no IgA response.
Sub. 84 showed both elevated and similar levels of IgG and IgA throughout.
In general, IgA and IgM responses appeared to decline while IgG responses tended to hold steady across the given timeframe.
half-life of IgA (time until the antibody concentration is half the original concentration) had a median value of 8.8 days vs. the half-life of IgM of 10.4 days.
Interestingly in one vaccinated individual (Sub. 83) vaccination appeared to have elevated all 3 classes of Ig.
Note that these results were from serum samples and don’t provide any information on the intranasal environment, so consider this one limitation of these results as the extent of IgA/IgG responses may differ in mucosal membranes.
However, it’s interesting that the researchers were able to detect an appreciable level of IgA antibodies. Several studies have suggested that early IgA responses may be a predictor of severe SARS-COV2, and may provide greater neutralization capabilities compared to IgG-mediated neutralization.7
This would be somewhat contradicted by Sub. 48 who appears to show hardly any IgA response. The researchers report that all participants showed typical symptoms of COVID, but they did not outline the individual severity among participants, meaning that there’s no evidence as to the severity of the symptoms Sub. 48 experienced.
However, these results are one of the first results to actually map the different humoral responses to infection. Although the sample size is limited, it shows just how varied the response to infection could be across individuals.
Case of Asymptomatic COVID
Interestingly, one participant (Sub. 82) presented with a positive case of SARS-COV2 as determined by PCR but didn’t present with any symptoms. The results are interesting and provides a much different picture compared to the above figures:
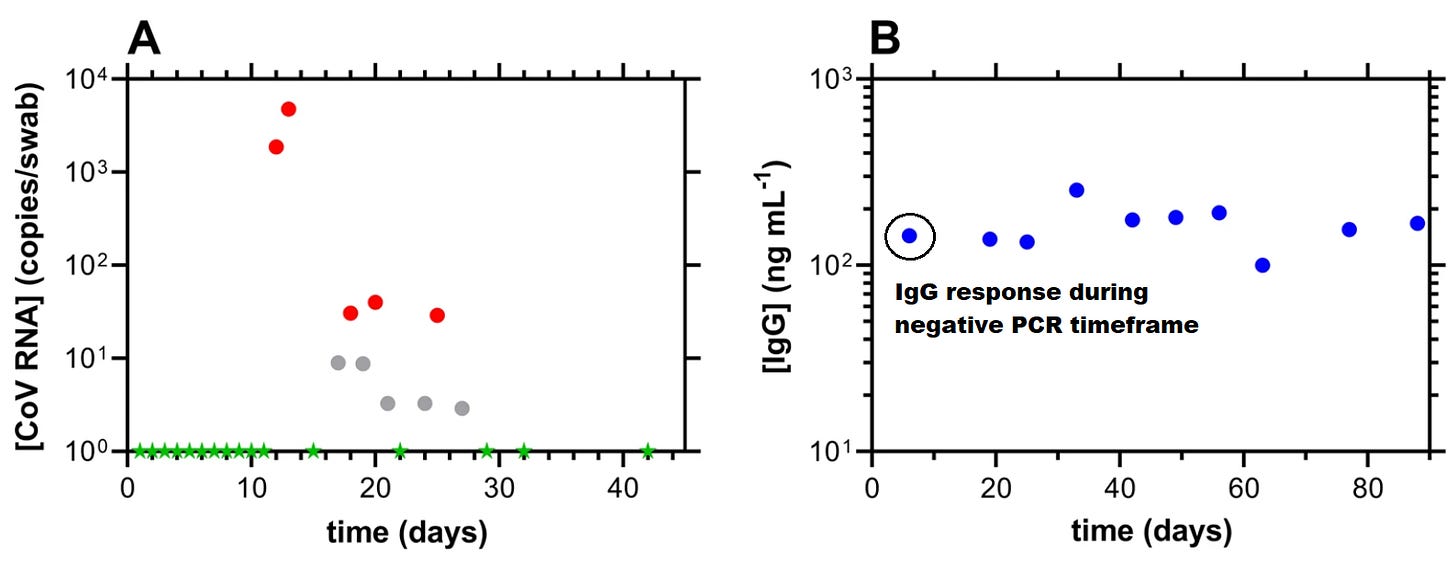
Note that very few nasal samples became positive and only an IgG response appears to have occurred. Interestingly, there appears to have been IgG antibodies present during the time in which the participant tested negative, and the positive IgG response appears to remain stable across the tested timeframe.
This would probably be one case in which humoral surveillance of the nasal passage may have provided critical insights, although that would have to be scrutinized by the negative PCR results with samples taken from the nose.
The researchers do note that two of Sub. 82’s household members had mild cases of COVID and may have been the reason for the asymptomatic infection. However, the evidence of IgG antibodies appearing during the negative PCR timeframe may be indicative of some prior unknown exposure that may have provided prior protection:
However, serum IgG concentrations were constant between ca. 100–200 ng mL−1 (Fig. 7), even preceding the first positive test result, indicating that the unvaccinated participant had acquired some level of immunity from previous, unknown exposure.
Cellular Response after possible SARS-COV2 Exposure
Lastly, researchers looked at the CD8+ T-Cell response of participants who either became positive or remained negative during the timeframe between March 23, 2020 - April 1, 2021, as determined by PCR results.
Blood was taken from both groups on April 1, 2021 and were challenged against peptide pools. Peptide pools are small amino acid fragments found on a given viral protein. For instance, 12 peptides from the spike protein were used to form the spike peptide pool in this study while only one peptide from the envelope protein was used.
If you can recall, this methodology was the same as the Immune Imprinting study that used the transgenic mice carrying the DRB*04:01 allele.
The results for the PCR positive participants are very interesting:
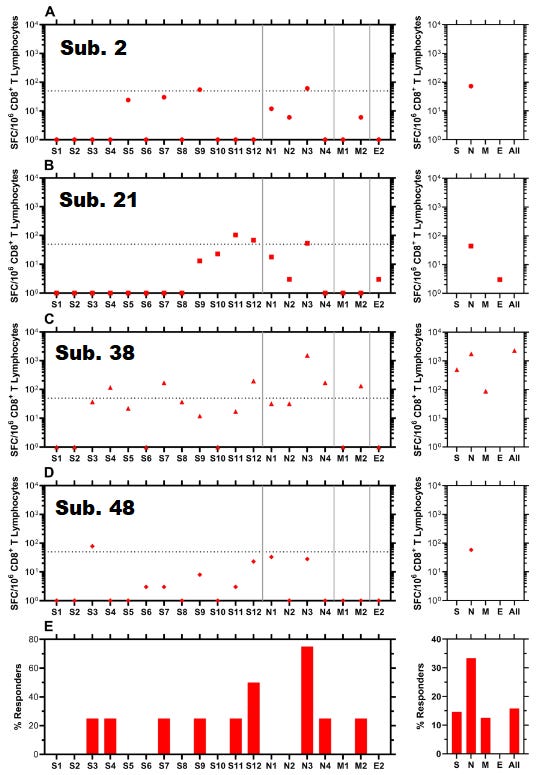
The left charts show frequency of T-cell response for each individual peptide from the corresponding peptide pool, while the right charts shows the total responses for each peptide pool (the researchers state that the right charts aren’t sums of the individual peptide pools as negative values were used to eliminate background noise). Horizontal lines show the cutoff for positivity while vertical lines divide the peptide pools into 4 distinct categories.
Panel E shows the % of responders to each peptide as noted by the number of samples that passed the horizontal line.
As can be seen here the T-cell response varied greatly between participants:
Most participants had T-cell responses to Nucleocapsid peptide pools
Spike and Matrix show a similar frequency of response while no response was shown for the Envelope peptide.
Sub. 48 showed limited response, with only one noted response to peptide S3 from the S peptide pool meeting the threshold for positivity. Note that Sub 48 also did not have a robust humoral response compared to other participants.
In contrast, Sub. 38 showed the most varied response with a positive response to at least one peptide from each peptide pool (aside from E).
Unfortunately, this is one of those times where deeper demographic data would be vital to contextualizing these results.
Remember that I lamented the fact that the Immune Imprinting study only used mice with the DRB1*04:01 allele meaning we wouldn’t know the T-cell response for other DRB1 alleles as well as the other HLA genes.
It’s clear here that the response would be heavily dictated by each participant’s HLA alleles. Given that these results were so closely monitored it’s strange that the researchers never thought to sequence each participants genes to get their HLA profile which would provide some necessary insights.
Did Sub. 48 have limited T-cell response due to their HLA alleles? Is the unique HLA alleles for Sub. 38 indicative of a favorable protection against SARS-COV2 based on the varied T-cell response?
It’s a shame, because these results feel like the researchers got so close but just didn’t do that little bit of extra effort that would have been so important for these results.
In any case, the results here mimic that of both the humoral immunity and the innate immunity results- people are different, and they therefore respond differently to infections.
I’ll leave it at that for now. I’ll provide some concluding remarks and inferences in a later post.
If you enjoyed this post and other works please consider supporting me through a paid Substack subscription or through my Ko-fi. Any bit helps, and it encourages independent creators and journalists outside the mainstream.

Gunawardana, M., Webster, S., Rivera, S. et al. Early SARS-CoV-2 dynamics and immune responses in unvaccinated participants of an intensely sampled longitudinal surveillance study. Commun Med 2, 129 (2022). https://doi.org/10.1038/s43856-022-00195-4
The graphs were constructed using the following two equations.
ln(y)=ln(y0)+k⋅t
this equation provides for the rate constant, k, by plugging on known, collected values.
For instance, y0 (pronounced y-naught) is the viral load on the “initial” day of infection as indicated by the first positive day.
y here would be the viral load at a different timepoint, say one day (24) after y0, or maybe even 8 hours afterwards. This value is likely to be derived from the slope constructed by different timepoints rather than a separate timepoint on its own.
t is time between y0 and y in hours (remember that units need to be consistent here and given that Td is provided in hours we can assume that t is measured in hours in this equation).
Plugging in all of these known values should give the value for k, which can then be put into the next equation:
Td=ln(2)/k
The only value needed here would be the k value, which the first equation should find, and that should provide the Td value.
You can try with the slope created for subject 2 below to see if you get a similar Td value. Note that the y-axis for this graph is already a log function so you wouldn’t need to calculate the ln—just plug in the values from the slope below.
The equation also depends on the initial viral load, so even discrepancies among the first day of positivity will influence the doubling time since it may provide an incorrect starting point.
Note that more common cytokines such as IL-6 and IL-10 were not included in this study, and that appears to be due to the fact that these cytokines were not detectable in the given assays or were limited to only a select few participants:
Interleukin-1 alpha (IL-1α) and interleukin-2, −4, −6, −10, −13, −15, and −17A (IL-2, IL-4, IL-6, IL-10, IL-13, IL-15, and IL-17A) concentrations either were below the limit of quantification in most samples (IL-2, IL-6, and IL-15), or were only observed in a minor subset of samples.
Burak Pamukcu, Gregory Y. H. Lip, Viktor Snezhitskiy & Eduard Shantsila (2011) The CD40-CD40L system in cardiovascular disease, Annals of Medicine, 43:5, 331-340, DOI: 10.3109/07853890.2010.546362
Quinti, I., Mortari, E. P., Fernandez Salinas, A., Milito, C., & Carsetti, R. (2021). IgA Antibodies and IgA Deficiency in SARS-CoV-2 Infection. Frontiers in cellular and infection microbiology, 11, 655896. https://doi.org/10.3389/fcimb.2021.655896
Very interesting article- this is the sort of data we ought to have by now. It is a good start and you have raised several great follow-ups to this study.
Overall the data does show a ton of variability between subjects which totally illustrates the complexity of the immune system & the differences between our immune statuses (health of our immune systems at the time of infection). It seems like most things are presented by the powers that be as being black & white, but immunology is nothing but gray!
So interesting! My sister-in-law is a primary care dr who has never contracted covid despite her family getting it and of course seeing tons of pts. She is vxd obviously but attributes her lack of covid to repeated exposure, like Sub. 82 whose family had covid but was asymptomatic herself. Immunology is fascinating!